Medical Research Publishing Needs New Metrics
Open access and preprint articles are overwhelming the industry with research; we need a more trustworthy, explainable and significant way to separate the high quality data from low quality data
I have been in the healthcare field for a couple of decades now; long enough to see a lot of changes. Faxes replaced by email, paper replaced by electronic healthcare records, office consults replaced by smartphone apps. But nowhere has there been as much change as in medical and scientific research publishing.
As recently as 2009 research publishing was mostly the purview of a relatively small group of academic publishers controlling the majority of journals. As gatekeepers of information, the lifeblood of science, these journals were able to generate substantial revenues through both fees to subscribe to more prestigious publications, and fees to publish (known as author publishing charges or APCs) in lower impact partner publications.
The Rise of Open Access
Things began to change in 2004. The U.S. National Institutes of Health (NIH) Public Access Policy mandated open access to research they funded, and Europe’s Plan S soon followed suit. Digital publishing and Internet access made widespread electronic distribution possible; open access and preprint publishing platforms soon followed, opening the research access floodgates.
In The Changing Medical Publishing Industry: Economics, Expansion, and Equity, a paper published by a group of physicians in the Journal of General Internal Medicine (JGIM) in July of 2023, the authors note the number of open access journals over the past 20 years has risen from near zero to over 16,000.
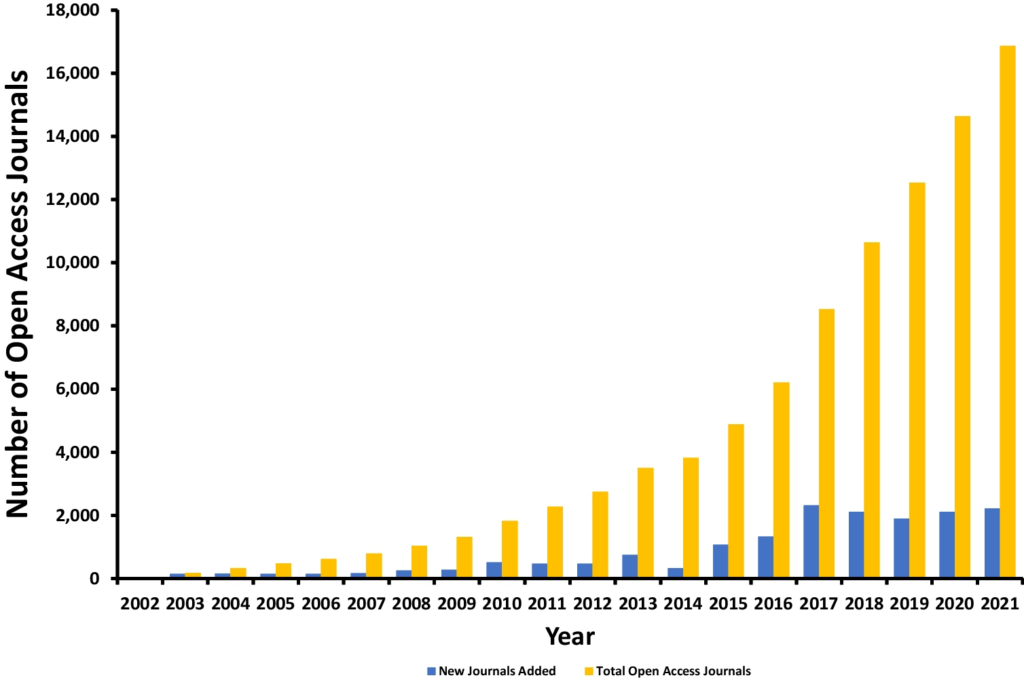
Source: The Changing Medical Publishing Industry: Economics, Expansion, and Equity, Springer.com
The authors note that we are only beginning to understand the opportunities, and consequences, today.
…open access will result in wider dissemination, and preprint platforms with faster communication of science… However, consumers of research may have more difficulty assessing the validity of conclusions from the increased number of research reports… Rapid expansion of published research brings with it the risks of prioritizing quantity over quality of medical research. This poses risks to patients if clinical decisions are influenced by shoddy science.
Identifying Quality Among Quantity
Of course, quantity over quality has always been an issue in scientific research, which is why there are checks like conflict disclosures, statements of limitations, the h-index, and peer review. But even with these, sheer volume and velocity of newly published research, and the opaque nature of peer review makes the task of identifying valuable knowledge in a rising sea of information extremely difficult. The JGIM paper’s authors note that current methods are better than nothing, but still a far from a complete solution:
“…peer review remains an important part of the scientific method. We acknowledge that the empiric benefits of peer review remain debatable, and it may not filter high-quality from low-quality research as well as we like to believe. However, we believe it is better than an alternative system in which there is no formal process for peer input and constructive critique.”
The writers go on to suggest that newer open models including engagement via comments on preprint platforms and community peer review via systems like PubPeer have potential. However, my own experience with healthcare providers, researchers and other consumers of this information, indicates that inputs like these will probably fall short. They’re labor-intensive and, more importantly, as we’ve seen in other open discussion platforms, subject to manipulation by bad actors and robots. As a result, for the Open Access research model to succeed, new models for vetting and valuing research will also be required.
Developing New Metrics for Research Quality
One of the biggest problems with open access and preprint literature is also one of the oldest: “information overload.” Researchers at Oxford University define this as:
…the situation that arises when there is so much relevant and potentially useful information available that it becomes a hindrance rather than a help.
They note that this overload creates a condition creatively called data smog, which is a cloudy, unhealthy, perception of what information is actually available and whether it is of value. Anyone who has ever done a web search on a topic that seems fairly specific and straightforward only to be presented with a million results knows the data smog effect and the skepticism in any results it leads to.
Commercial search engines like Google have developed sophisticated models that do a pretty good job at identifying and presenting relevant information in search. Unfortunately, people have developed techniques in “search engine optimization” (SEO), to game search results in such a way that unless you are a sophisticated search engine user familiar with the commands and parameters for creating highly specific search queries, you are still likely to be showered with mostly irrelevant information.
The question is then, if a near-trillion-dollar search engine giant can’t create a reliable method for identifying and presenting high quality, trustworthy information, how is a frontier community like open access academic publishing supposed to?
Trust, Explainability, and Significance
Fortunately, we have examples of how this might be accomplished from applications in other scientific fields. Researchers are leveraging advances in data science, neural networking, machine learning, and to a lesser degree, artificial intelligence to successfully identify solutions for extremely complex problems. Rather than relying on Boolean parameters like AND, IF and NOT, they are using multi-modal analysis of vast quantities of information to develop models that identify and present the most promising data. Computer scientists working in these areas often refer to three very human terms—Trust, Explainability, and Significance (TES)—as the underpinnings of how they assist human beings in identifying high quality information in an otherwise limitless ocean of data.
TES is already being used to explore new avenues of research and help make incredible advances in a range of highly complex fields from molecular chemistry to financial market behavior analysis. Significantly, these fields have an open access history that is more agile and mature, but no less complex, than that of medical research publishing. As a result, leveraging TES-based technologies for both the discovery of high-quality information, and assisting human beings in confident decision-making based on it, offers considerable promise in healthcare research and clinical decision-making.
Traditional methods for establishing the validity and value of research will still have value in an open access, open distribution publishing model. But, without some means of separating the high quality from the low in an industry that is doubling the amount of available information every three months, information overload will grow, and confidence will fall. Incorporating Trust, Explainability, and Significance concepts into medical research exploration and search technologies offers a way to confidently advance and accelerate medical knowledge and improve healthcare delivery and outcomes.
Share This →
One Comment
Leave A Comment
Recent Posts
[…] many of the changes to scientific research publishing over the past decade have been positive, as I’ve noted before, there are plenty of people willing to game the system for their own advantage. We were reminded of […]