Why We’re Not All In With AI (Yet)
Artificial Intelligence has tremendous potential, but there’s a long way to go between what might be possible one day and what is real today.
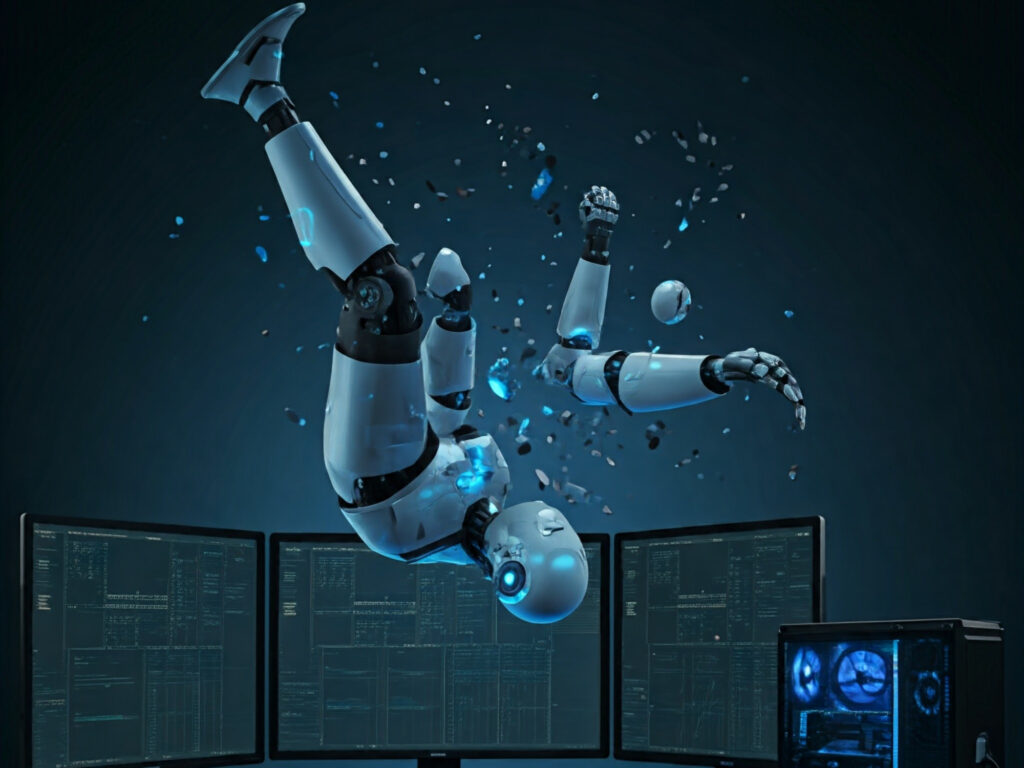
AI isn’t quite up to the task yet
As the founder of a tech startup, I spend a lot of time talking about my company and the problem it’s trying to solve. Naturally, because artificial intelligence is such a hot topic these days, people assume that it’s a big part of the “magic” in our platform’s technology.
The truth is though, AI only plays a minor role. It’s not that it isn’t a useful technology, it’s just that, despite what the hype is telling you, it’s not a good solution for every type of problem. Actually, it’s not a good solution for most problems, particularly the complex ones in medical and scientific research we’re trying to solve.
Even for those problems that AI is good at, it still has a long way to go before the real value of AI matches the hype. Here are the key weak spots we see in AI today:
AI promises are overblown
Despite the high-profile entrepreneurs trying to create a wave of excitement and raise money to pursue their ventures, most of the promises and predictions are really based on hopeful thinking rather than reality.
Yes, it would be nice if AIs were like Tony Stark/Iron Man’s J.A.R.V.I.S. – conversational, fast, and 100% accurate. But the reality is, today’s AIs, even after extensive training, might get to 90% accuracy… most of the time. With additional, highly focused training, it might get to 95%. But that last 5% will probably never happen.
Admittedly, 95% is pretty good if you’re working on a 5th grader’s book report, or trying to learn how many bluefin tuna are caught off the coast of Mexico each year. But when it comes to subjects where “good enough” isn’t – and there are all kinds, ranging from aircraft design to life-saving biopharma research – it’s nothing you’d want to trust.
AI is power hungry and expensive
Finally, AI is incredibly expensive. Running an AI requires immense computational resources which, in turn, requires immense amounts of electricity to power, heat and cool the hardware. Even big tech companies like OpenAI, Microsoft, and Meta publicly admit those costs are so high that their AI initiatives struggle to be profitable. Additionally, the more complex the AI, the more expensive it is to run.
Typically, we would expect the costs of technology to decline over time. However, the real costs of AI aren’t in the software or hardware, they are in the power and bandwidth it takes to run it. Demand for AI is so high that global data center capacity, which will double over the next five years, will still lag demand. Moreover, electricity consumption is expected to increase almost 25% a year through the end of the decade. That double-whammy is expected to keep prices sky high through at least the end of the decade.
AI isn’t good at most tasks
Beyond the hype problem and expense, there are simply many kinds of real-world problems that AI just can’t solve.
A lot of the appeal in AI is the idea that it’s some sort of super smart Star Trek computer that can answer questions and solve problems better, faster and cheaper than a human. However, AI isn’t actually “intelligent”, it’s just a really big statistical analysis system. It’s very good at giving you the most likely answer based on a statistical match with the questions you ask it, but it’s completely useless when it comes to “understanding” the question.
For example, if you show a person a “no trespassing” hanging upside down and ask them “can you fix that?” the person will have no problem recognizing that the sign is upside down and turn it right side up. An AI on the other hand, unless it has been specifically trained on that upside down imagery, and that for it to be proper it must be turned 180 degrees, will fail every time.
In other words, AIs can’t reason or do novel problem solving the way people can, so their use is limited to repeating that which has already been done. Again, this is fine for the 5th grade book report on Moby Dick, but when it comes to finding novel solutions or answering questions that haven’t already been answered and documented, AI is neither better nor cheaper than a human being.
Where does AI fit?
Having listed a number of ways AI isn’t the end-all-be-all technology, I should note where AI does have its uses.
As I mentioned above, AI can’t create anything that hasn’t been created before, but it is very good at (re)processing things that already exist to present them in a new way. For example, AI is very good at re-stating and summarizing longform written content. It’s also pretty good at identifying similarities and relationships among different content different pieces. And if you want to know what the pope would look like in a puffy Balenciaga jacket, AI is the way to go.
At Siensmetrica, we’re using AI to quickly deliver summaries of research papers, identify the points of consensus among distributed findings, and even help filter results among well-documented metrics like the h-index.
But when it comes down to diving deep into materials to identify novel or difficult-to-articulate measures of what makes the content of one thing more likely to be reliable than another, we’re relying on a whole series of inputs, multi-vector data analysis, and fuzzy weighting factors like trust, explainability and significance to do it.
Maybe one day AI will have the capabilities and the cost-benefit ratio that make it a viable all-in-one solution for the technology we’re developing. But until then, we’re not going all in on it.